
Variable Problem Dimensionality in Evolutionary Computation (VarDimInEC)
It’s been a long time since MessyGA.
Description
In the realm of AI, addressing complex optimization challenges with variable problem dimensionality remains an underexplored area. Existing meta-heuristic algorithms excel in solving optimization problems but often struggle when faced with problems of changing dimensionality. By addressing this critical research gap, the research findings not only advance the field of AI-driven optimization but also offer practical solutions to enhance the performance and applicability of meta-heuristic algorithms in dynamic and uncertain environments. The results and methodology may have significant implications for a wide range of domains, such as network design, resource allocation, and machine learning, where variable problem dimensionality is prevalent and challenging.
This special session aims to bridge this gap by discussing the original research focused on adaptive strategies for meta-heuristic optimization tailored to variable problem dimensionality. Such research may become a vitally important part of science and engineering at the theoretical and practical levels. The research papers presenting the extensive analysis of the proposed methods, using both benchmark problems and real-world applications, as well as discussing the research focusing on evaluating the adaptability of these algorithms, comparing their performance, and assessing the trade-offs between algorithm complexity and adaptability, are welcomed.
Our aim with this special session is to bring together experts from fundamental research of evolutionary computation and experts from various evolutionary computation application fields researching and developing novel algorithmic approaches capable of dynamically adjusting search operators, population sizes, and exploration-exploitation strategies in response to variations in problem dimensionality. The scope will be focused on, but not limited to, the following topics.
List of topics
- Applications of nature-inspired computing and optimization algorithms for problems with variable dimensionality.
- The performance improvement, benchmarking, testing and efficiency of the proposed algorithms.
- Creation of new benchmarking sets.
- Adaptive strategies for meta-heuristic optimization tailored to variable problem dimensionality.
- Visualizations and methodology for analysis of population dynamics with variable dimensionality problems.
- Adjusting state-of-the-art meta-heuristic techniques for global optimization to the problems with variable dimensionality.
Organizers
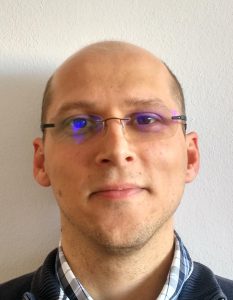
Researcher
|
Assoc. Prof. Michal Pluhacek Ph.D.
Regional Research Centre CEBIA-Tech
Head of the swarm intelligence research group
Contacts:
Research Focus:
- Swarm Intelligence
- Particle Swarm Optimization
- Adaptive Techniques for Swarm Algorithms
- Swarm Robotics
|
Short Bio
Assoc. prof Michal Pluhacek received his Ph.D. degree in Information Technologies in 2016 with the dissertation topic: Modern method of development and modifications of evolutionary computational techniques. He became an assoc. prof. in 2023 after successfully defending his habilitation thesis on the topic „Inner Dynamics of Evolutionary Computation Techniques: Meaning for Practice.“ He currently works as a senior researcher at the Regional Research Centre CEBIA-Tech of Tomas Bata University in Zlin, Czech Republic. He is the author of many journal and conference papers on Particle Swarm Optimization and related topics. His research focus includes swarm intelligence theory and applications and artificial intelligence in general. In 2019, he finished six-months long research stay at New Jersey Institute of Technology, USA, focusing on swarm intelligence and swarm robotics. Recently, he is focusing his research on the interconnection of evolutionary computing and the large language models.
|
|
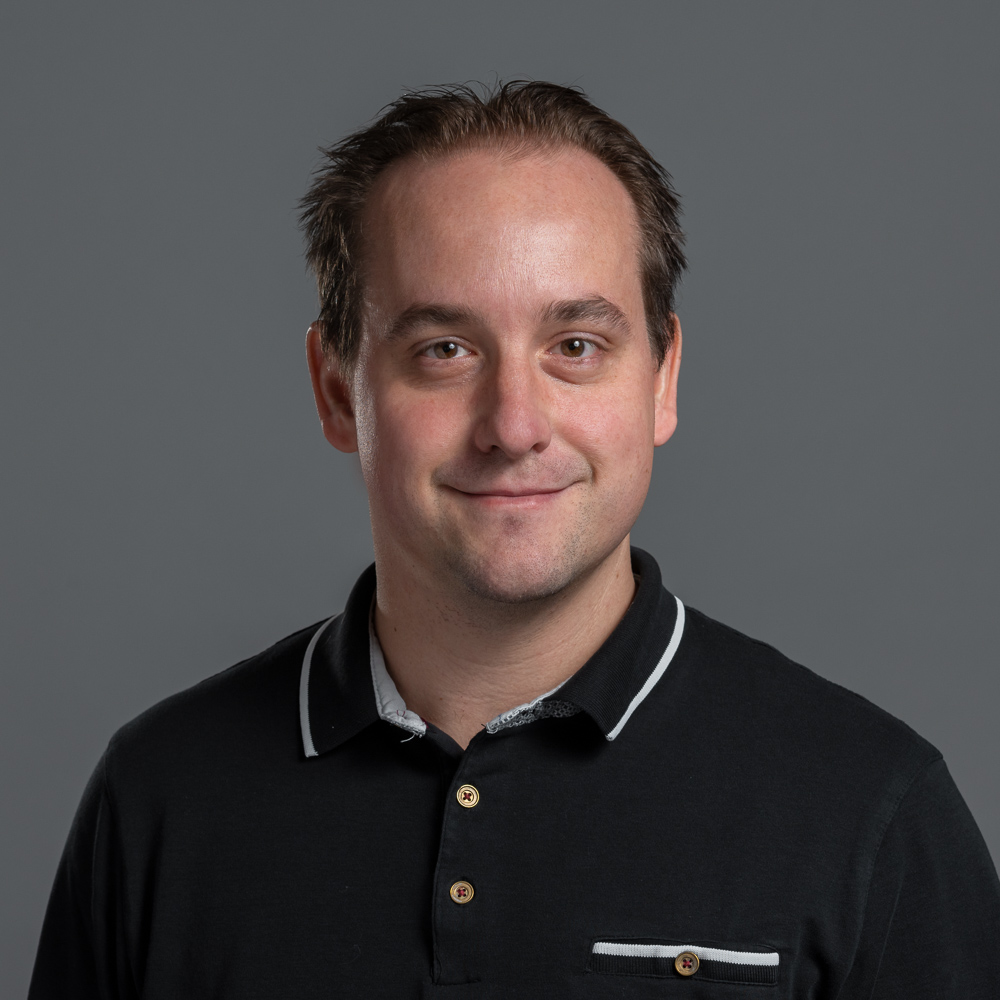
Head of the lab
|
Prof. Roman Senkerik Ph.D.
Department of Informatics and Artificial Intelligence
Head of the evolutionary computing research group
Contacts:
Research Focus:
- Computational Intelligence
- Evolutionary Algorithms
- Optimization
- Chaos Theory
|
Short Bio
Roman Senkerik was born in Zlin, the Czech Republic, in 1981. From 2008 to 2013 he was a Research Assistant and Lecturer with the Tomas Bata University in Zlin, Faculty of applied informatics. Since 2014 he is an Associate Professor and since 2018 Head of the A.I.Lab https://ailab.fai.utb.cz/ with the Department of Informatics and Artificial Intelligence, Tomas Bata University in Zlin. He is the author of more than 50 journal papers, 250 conference papers, and several book chapters as well as editorial notes. His research interests are the development of evolutionary algorithms, their modifications and benchmarking, soft computing methods, and their interdisciplinary applications in optimization and cyber-security, machine learning, neuro-evolution, data science, the theory of chaos, and complex systems. He is a recognized reviewer for many leading journals in computer science/computational intelligence. He was a part of the organizing teams for special sessions/workshops/symposiums at GECCO, IEEE WCCI, CEC, or SSCI events.
|
|
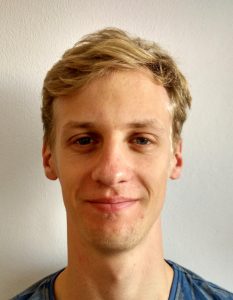
Lecturer / Researcher
|
Ing. Adam Viktorin Ph.D.
Department of Informatics and Artificial Intelligence
Contacts:
Research Focus:
- Artificial Intelligence
- Adaptive strategies for Differential Evolution
- Running around
|
Short Bio
Adam Viktorin was born in the Czech Republic in 1989, and went to the Faculty of Applied Informatics at Tomas Bata University in Zlín, where he studied Computer and Communication Systems and obtained his MSc degree in 2015. He obtained his Ph.D. degree at the same University in 2021 with the thesis topic: Control Parameter Adaptation in Differential Evolution. Among his professional interests are: development and analysis of adaptive strategies for Differential Evolution in the area of numerical optimization; and also application of such algorithms to real-world problems.
|
|